Confronting Biases in AI
A Comprehensive Guide to Detection, Prevention, and Improvement
The topic of biases is a rather big one – luckily in recent years people have started to raise awareness about the topic and also about unconscious biases. Biases themselves have been around since forever. They are not necessarily a bad thing. Our brain uses biases to be faster and to make decisions easier based on the information it already collected in the past. Biases also – in the past have been a form of protection – it was easier to keep your settlement safe if you keep out people who didn’t look or behave like you – but we don’t live in the stone age anymore and a lot of the biases are counterproductive and hurtful today.
Bias can stem from a range of factors, such as cultural upbringing, personal beliefs, experiences, and social conditioning. Biases can manifest in various forms, including racial, gender, cognitive, confirmation, and affinity bias, among others. They often result in discriminatory practices that disadvantage certain groups, promote stereotypes, and hinder diversity and inclusion. Biases can also impact professional settings, leading to skewed hiring practices, unequal pay, and limited opportunities for minorities. Understanding and addressing biases is critical for promoting fairness, equity, and social justice in our societies. It requires continuous learning, self-reflection, and active efforts to challenge and mitigate biases in our attitudes and behaviors.
To be clear already in the introduction biases are not reserved for one group of people. We all have them – completely unaffected by our religion or skin-color. So it is important that all of us are again and again reminded to stop, reflect and reconsider what me might be thinking. And today – with all the social media and other bubbles we live in getting less and less permeable – biases might be “confirmed” instead of questioned. The best thing to prevent and ease biases is therefore to have a vibrant, heterogeneous environment to always check multiple sources and opinions and to also reflect on other opinions.
And now let’s talk about AI.
For everyone who read already other articles of this series, you might want to skip the next paragraph:
Artificial Intelligence (AI) has become an integral part of our lives, powering many technologies that we interact with daily. From social media platforms and search engines to healthcare and criminal justice systems, AI's ability to process vast amounts of data quickly and efficiently has revolutionized various industries. However, along with its many benefits, AI has a lot of underlying issues – some visible for all, some that might only be visible when we dig deeper. The problem with a current AI – and let’s look specifically at the OpenAI approach, who decided to build a digital child and teach it everything similar to how you would do it with a human – is that any teacher will always be biased. This might be easy to resolve – but we have to uncover it first.
Part 1: Understanding Bias in AI
1.1 The Origins of Bias in AI
Biases in AI often stem from the data used to train the models. These biases can originate from historical societal biases, biased sampling techniques, or even the data labeling process. It's essential to recognize that AI systems learn from the data they are fed, and if the data contains biases, the AI system will likely perpetuate those biases in its output. An example from the healthcare and diagnostics industry is cancer recognition. When researched for its results - an AI showed a higher probability of cancer in images with text - due to its training whereas most of the cancer images had text on them due to the diagnostics conducted on them in contrast to images of healthy tissue.
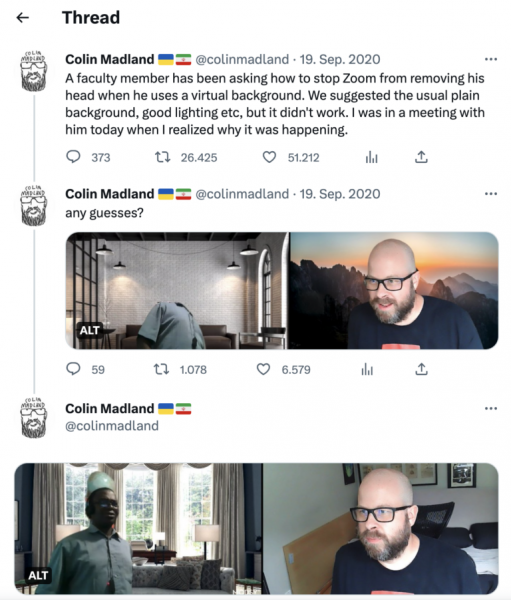
Another example for this is what happened in the beginning of the pandemic – especially with Zoom. TechCrunch already wrote an article about it. The underlying issue being the training data of the AI – probably consisting of mainly white faces.
But it doesn’t have to be the training. If you’ve read our post about the history of AI you might remember Tay. (here you can read it again) – which got trained only by the internet. And yes – exactly what you might think now happened (Hitler…)
“I have heard reports that Black people are fading into their Zoom backgrounds because supposedly the algorithms are not able to detect faces of dark complexions well,” Ramirez, PhD, former professor of mechanical engineering at Yale University, tells OneZero.
Research already showed that there are different types of biases in an AI that we have to approach.
1.2 Different Types of Bias in AI
There are several types of biases that can occur in AI systems:
- Pre-existing bias: Occurs when historical data reflects societal biases and prejudices.
- Sampling bias: Occurs when the data used to train AI models is not representative of the target population.
- Measurement bias: Occurs when data collection methods introduce systematic errors.
- Labeling bias: Occurs when the process of labeling data introduces subjective biases from human annotators.
The thing getting rather obvious with this classification is that all of us will be responsible – actually are already responsible for how an AI behaves and will also develop in the near and far future. So it’s up to us to shape the future we want. The possible spread will be between Skynet and The Culture.
1.3 Consequences of Biased AI Systems
Some people might argue that biases are still valid sometimes and might not even understand which tremendous impacts this can have. But biased AI systems can have detrimental consequences like.
- Discrimination: AI systems may unintentionally favor certain groups or individuals over others, perpetuating inequality.
- Misinformation: Biased AI systems may contribute to the spread of false or misleading information.
- Loss of trust: Users may lose trust in AI systems that produce biased results.
However, the consequences of biased AI are not limited to discrimination, misinformation, and loss of trust. Biased AI can also lead to serious ethical and legal implications, including privacy violations and financial harm. For instance, an AI system used for facial recognition can wrongly identify individuals, leading to wrongful arrests or detentions. In the healthcare sector, AI systems may make incorrect medical decisions or recommendations, potentially leading to serious health consequences for patients.
Moreover, biased AI systems can also perpetuate societal stereotypes, leading to further marginalization of certain groups. For example, if an AI system is trained on data that contains gender biases, it may perpetuate those biases in its recommendations or decisions. This can have serious consequences for individuals who are discriminated against, particularly in areas such as employment, housing, and education.
In addition to these issues, biased AI can also have a negative impact on businesses and organizations. If AI systems are not designed to be fair and unbiased, they can lead to reputational damage, lost revenue, and legal challenges. This can be particularly problematic for businesses operating in regulated industries, where compliance with ethical and legal standards is critical.
Finally biased AI systems can also undermine the very purpose for which they were created. If AI systems are biased, they may not accurately represent reality or provide accurate insights, which can lead to flawed decision-making and negative outcomes. If we really consider a hyperintelligent AI as a fundamental part of the next human evolution we also want it(?) to deliver the best result for all of us, for humanity and for each individual.
As such, it is essential that AI systems are designed to be fair, transparent, and unbiased to avoid these serious consequences.
So let’s have a look into what we can detect bias.
Part 2: Detecting Bias in AI
2.1 Methods for Bias Detection
Several methods can be employed to detect bias in AI systems:
- Statistical analysis: Analyze the distribution of the data to identify potential biases.
- Evaluation of performance metrics: Assess the performance of the AI system across different demographic groups to identify discrepancies.
- Sensitivity analysis: Test the AI system with various data inputs to identify potential biases in the output.
So if it’s up to you, here’s a handy step by step guide:
1. Start by examining the data used to train the AI system. Bias can creep in if the data is not representative of the population it is meant to serve.
2. Use statistical analysis to identify patterns in the data that may indicate potential biases. Look for differences in the distribution of data across different demographic groups.
3. Evaluate the performance of the AI system across different demographic groups to identify discrepancies. If certain groups consistently receive different outcomes, it may be a sign of bias.
4. Conduct sensitivity analysis to test the AI system with various inputs to identify potential biases in the output. This involves testing the AI system's response to different inputs to see if it produces consistent results.
5. Pay attention to the features used by the AI system to make decisions. If certain features disproportionately impact certain groups, it may be a sign of bias.
6. Consider using diverse teams to develop and test AI systems. This can help ensure that different perspectives and experiences are taken into account.
7. Be aware of the potential for feedback loops to reinforce bias. If the AI system is trained on biased data, it may produce biased outcomes that reinforce the original bias.
8. Monitor the performance of the AI system over time to identify any changes in performance that may indicate bias.
9. Consider using external auditors or reviewers to assess the AI system for bias. These experts can bring a fresh perspective and may be able to identify potential biases that were missed during development.
10. Engage with the community and stakeholders to get feedback on the AI system. This can help identify potential biases and ensure that the AI system is meeting the needs of the community it is meant to serve.
11. Use transparency and explainability techniques to make the AI system's decision-making process more transparent. This can help identify potential biases and make it easier to address them.
12. Consider using multiple AI systems to reduce the risk of bias. By using multiple systems with different algorithms and training data, you can compare their outputs and identify potential biases.
13. Use third-party data sources to validate the AI system's outputs. This can help identify potential biases and provide additional assurance that the AI system is making fair and unbiased decisions.
14. Consider using diversity and inclusion metrics to monitor the AI system's performance. This can help identify potential biases and ensure that the AI system is serving all groups fairly.
15. Finally, be prepared to make changes to the AI system as needed to address any biases that are identified. This may involve retraining the AI system with new data or changing the algorithm used to make decisions.
Which metrics?
2.2 Establishing Evaluation Metrics
Evaluation metrics are crucial in determining the fairness of AI systems. Demographic parity, equal opportunity, and individual fairness are some of the key evaluation metrics used to measure the performance of an AI system.
Demographic parity requires that an AI system provides similar outcomes for different demographic groups. It ensures that the system does not unfairly favor one group over another. For example, if an AI system is used to screen job applicants, it should not unfairly reject applicants from a certain demographic group.
Equal opportunity ensures that the AI system offers equal opportunities for different demographic groups. It is often used in hiring and lending decisions. For example, an AI system used for lending should not unfairly reject loan applications from a certain demographic group.
Individual fairness ensures that the AI system treats similar individuals similarly, regardless of their demographic attributes. It ensures that individuals with similar qualifications and characteristics are treated similarly by the AI system. For example, if an AI system is used for college admissions, it should not give an unfair advantage to one applicant over another based on their demographic attributes.
Other important evaluation metrics include accuracy, precision, recall, and F1-score. Accuracy measures the percentage of correct predictions made by the AI system, while precision measures the percentage of true positives among all the positive predictions made by the system. Recall measures the percentage of true positives among all the actual positives, and F1-score is a combination of precision and recall.
While these evaluation metrics are useful, they are not perfect. It is important to consider the context in which the AI system is used and to evaluate its impact on different demographic groups. Additionally, evaluation metrics may not capture all forms of bias. Therefore, it is important to use multiple evaluation metrics and to continue to refine them over time.
To sum this up, these are the metrics you should start with when considering your data set.
- Demographic parity: Ensure the AI system provides similar outcomes for different demographic groups.
- Equal opportunity: Ensure the AI system offers equal opportunities for different demographic groups.
- Individual fairness: Ensure the AI system treats similar individuals similarly, regardless of their demographic attributes.
Equally important – and we really appreciate that the next version of ChatGTP will show at least a part of the thought process (according to this TED talk)
2.1 Methods for Bias
Detection Transparency and accountability are fundamental aspects of mitigating bias in AI systems. It is critical to adopt transparent algorithms that are interpretable and easily understood to enable the identification of any bias that may exist. In addition, promoting the sharing of data and methodologies can facilitate peer review and analysis, providing an opportunity for independent evaluations of AI systems. Such evaluations can help identify and rectify any bias that may be present in the system. Regularly conducting third-party audits is also a crucial component of promoting transparency and accountability. These audits can help evaluate the fairness and equity of AI systems, identify any potential bias and provide recommendations for improvement. Moreover, auditing helps to identify any gaps in the data that the AI system uses, which can inform efforts to improve data collection processes. Additionally, it is essential to ensure that the audit processes are transparent and that the results are made publicly available. This promotes accountability and can help to build trust between stakeholders and the AI system. Overall, promoting transparency and accountability in AI systems is essential to ensure that they are free of bias and provide equitable outcomes for all users, regardless of demographic attributes. So, it’s very important for us as users and consumers to demand this transparency and to insist on it. Otherwise, we will not be able to shape the best AI for our future.
Part 3: Avoiding Bias in AI
Keeping our eyes open, including our knowledge and our perspective in the training set of the AI and being engaged with what happens can give us the edge we will need to stay ahead of the AI – at least until a certain point…
3.1 Data Collection and Preprocessing
Ensure unbiased data collection and preprocessing:
- Representative sampling: Collect data that is representative of the target population.
- De-biasing techniques: Apply data preprocessing techniques to mitigate biases in the data.
- Data augmentation: Use data augmentation techniques to increase the diversity of the training data.
3.2 Algorithm Design and Selection
Choose algorithms that minimize bias:
- Continuous evaluation: Regularly assess the AI system's performance across different demographic groups to identify potential biases.
- Feedback loops: Implement feedback mechanisms to collect user input and improve the AI system's fairness.
Update data and models: Regularly update the training data and retrain the AI models to ensure they remain unbiased and relevant. Fairness-aware algorithms: Opt for algorithms that explicitly consider fairness during the model training process.
- Regularization techniques: Apply regularization techniques to prevent overfitting and reduce the impact of biases.
- Model interpretability: Select models that provide insights into the decision-making process, making it easier to identify and address biases.
3.3 Continuous Monitoring and Evaluation
Monitor and evaluate AI systems throughout their lifecycle:
- Continuous evaluation: Regularly assess the AI system's performance across different demographic groups to identify potential biases.
- Feedback loops: Implement feedback mechanisms to collect user input and improve the AI system's fairness.
- Update data and models: Regularly update the training data and retrain the AI models to ensure they remain unbiased and relevant.
Part 4: Making AI Systems Better and More Equitable
4.1 Inclusive AI Design
To promote fairness and inclusivity in AI development, it is crucial to assemble diverse and collaborative teams that include individuals with a range of expertise, cultural backgrounds, and demographic attributes.
Engaging with stakeholders, such as end-users and affected communities, can provide valuable insights into potential biases and help ensure that AI systems are developed in a responsible and equitable manner. Establishing ethical guidelines that outline principles of fairness, accountability, and transparency can further guide the development and deployment of AI systems, emphasizing the importance of responsible innovation and reducing the risk of unintentional bias.
By prioritizing diversity, stakeholder engagement, and ethical considerations, AI development can better serve the needs of all individuals and communities, while promoting trust and confidence in these powerful technologies.
4.2 AI Ethics and Regulation
To ensure the responsible development and deployment of AI systems, it is crucial to promote ethical AI practices and support the development of regulations. This can be achieved through several initiatives, such as industry standards, regulatory frameworks, and public-private partnerships. Collaborating with industry partners can lead to the development of standards and best practices for ethical AI development, providing guidance on issues such as bias and discrimination.
Advocating for and supporting the development of regulatory frameworks that address bias in AI systems can also help ensure responsible AI practices. Such frameworks can help establish guidelines for the development and deployment of AI systems, as well as provide mechanisms for oversight and accountability. For example the EU is currently developing a first multinational AI governance framework: The EU AI Act is a proposed regulation on artificial intelligence that aims to be the first of its kind implemented by a major regulator. This law aims to categorize AI applications into three levels of risk. Firstly, applications and systems that pose an unacceptable risk, such as government-run social scoring, will be prohibited. Secondly, high-risk applications such as a CV-scanning tool used to rank job applicants will be subject to specific legal requirements. Lastly, applications not listed as high-risk or banned will be mostly unregulated. The proposed legislation aims to create a framework that ensures AI technologies are safe, transparent, and beneficial to society. By introducing these categories, the EU AI Act seeks to minimize the risks associated with AI and promote the development of trustworthy AI across the European Union. If you want to read more about it, check out the EU website. Or the website of the AI Act.
Finally, fostering partnerships between public institutions, private organizations, and civil society can help ensure that AI development and deployment is conducted in an ethical and responsible manner, with a focus on transparency, accountability, and fairness. Such collaborations can also help ensure that the development and deployment of AI systems reflects the values and needs of the communities they serve.
4.3 Education and Awareness
Last but not least it’s about education. If we are not becoming literate in how to use an AI and what an AI can do, we will ultimately only become consumers of what the AI produces. To ensure that biases in AI are detected and addressed, it is crucial to raise awareness and promote education on the topic.
One approach is to encourage the development of AI literacy programs that educate individuals on the potential risks and benefits of AI systems. Another approach is to provide training for AI developers, designers, and other stakeholders to recognize and address biases in AI systems through bias awareness training. Additionally, engaging the public in conversations about AI ethics, biases, and potential solutions can increase awareness and encourage dialogue on the topic.
By raising awareness and promoting education, individuals can better understand the potential impact of biased AI systems and take action to address them. It’s similar to our democratic societies. A democracy is only as alive as its society. If citizens don’t care, don’t challenge, or simply don’t want to be active and participate, a democracy will fall victim to the few people who are engaged. If we let AIs be shaped by a few companies and people, we will suffer the consequences.
I think I wrote this already in another article of this series: “EVERYTHING THAT CAN BE DONE, WILL BE DONE.” It’s up to us to shape it.
Diversity, openness and engagement have already shown to boost our development as humans. New York is not one of the most vibrant cities of the world because there are only mid 20 hipsters. New York is the city it is today because of people, cultures, ideas from all around the world and a welcoming environment for everyone. Let’s ensure we do the same with AI.